AN APPROACH TO THE STUDY OF IMPLEMENTAION OF KAZAKH SLANG DICTIONARY FOR BETTER SENTIMENT ANALYSIS IN KAZAKH
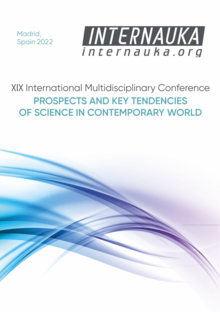
AN APPROACH TO THE STUDY OF IMPLEMENTAION OF KAZAKH SLANG DICTIONARY FOR BETTER SENTIMENT ANALYSIS IN KAZAKH
Dauren Rakhymzhanov
Master student, Kazakh British Technical University,
Kazakhstan, Almaty
I. ABSTRACT
Our life cannot be imagined without the internet or social networks. Everyday millions of people write comments or posts on the Social Network Sites (SNS). Thus, SNS can be viewed as an important place to get information about people’s opinions. Sentiment analysis refers to the task of identifying information related to the feelings and attitudes expressed in natural language texts. More and more persons extensively use informal language as slang in everyday discussions, social media, and mobile platforms and understanding the sentiment of slang helps in overall sentiment analysis. There are several works have been done in creating slang sentiment dictionaries for different languages, but not for the Kazakh language. In this paper, we would like to create a Kazakh sentiment slang dictionary from the online Kazakh slang dictionary website and it’s Instagram* page.
II. INTRODUCTION
Today people’s daily life cannot be imagined without Social Network Sites (SNS). Every day people of mixed ages give their reviews, comment on something, and express their opinions on social networks like Facebook*, Instagram*, VKontakte, Twitter*, etc. A large amount of information from various social networks stores valuable data. To get this valuable data from users, text sentiment analysis is often used. Sentiment analysis refers to the task of identifying opinions, favorability judgments, and other information related to the feelings and attitudes expressed in natural language texts. [3] Sentiment analysis distinguishes text into positive or negative for better understanding the polarities of people’s emotions and points of view. But because SNS is open platform users tend to use casual language in texting which includes a lot of neologisms and slangs that hard to find in the dictionaries. This process is complicated by the fact that nowadays many people on the Internet write in an informal language of communication. Slang used by people every day can also be considered as one of this non-formal language. More and more persons extensively use informal language as slang in everyday discussions, social media, and mobile platforms. Therefore, slang sentiment analysis is highly important for a better understanding of the polarity of the text and the information on the internet.
To better define the sentimentality of words, couple of dictionaries have been developed, including for the Kazakh language [11] [12]. But at the moment we are not aware or have not seen works on the creation of a slang dictionary for the Kazakh language. And since in many cases slang words are considered as noise(obstacle) for accurate analysis of the polarity of words they are usually removed in the process of preparing a suitable dictionary. We decided that the creation of a slang dictionary in the Kazakh language would be an essential contribution in the development of the field that works with text analysis in the Kazakh language. This is a difficult task because in most cases slang words are not included in regular dictionaries, and collecting words can be laborious. Another point is the determination of the sentiment
polarity, which takes a lot of time and effort. We would like to apply the method that [10] used to create the slang sentiment dictionary. To be more precise, we want to use web resources, like the recently created site for Kazakh slang words Jana Sozdik 1 for the first case. New words are added to this dictionary every day. In the second case, we want to find the sentiment of slang words by their description on the site.
III. RELATED WORK
There are several works to build a slang dictionary for different languages of the world including but not limited to English, Japanese, Arabian [1], and Indonesian [6].
For the English language was created SlangSD, where Wu etal. [10], created a slang sentiment dictionary for short phrases of the English language based on an Urban Dictionary, using the sentiment lexicons such as SentiWordNet , LIWC, MPQA as the first method for estimating sentiment strength. As for the second, the authors used a method that implies that words with a similar meaning often appear together. Twitter* data was taken for this purpose, as people often use slang on Twitter*. For the third option, they chose the list of related words that are provided in the description of the slang in the urban dictionary, because words with similar meanings might have the same sentiment polarity.
K. Manuel et. al [3] and others have made a system that 1)finds sentimental slang in the document selected by only subjective sentences 2) determines the polarity of each slang word in a sentence, and 3)determines the degree of slang polarity and the overall polarity of the sentence. Authors of this work computed polarity of the slang using Delta Term Frequency and Weighted Inverse Document Frequency technique with 5 levels of ratings from-2 to 2 [-2, -1, 0, 1, 2] where 2 is the review with a 5-star rating and -2 is the review with 1-star rating, respectively.
For the Japanese language, Matsumoto et al. [4] used Bag of Concepts to build a youth slang vocabulary with emotion. They used the word2vec tool to vectorize unknown words, as well as the k-nearest neighbor method and the maximum entropy
method based on Bag of Concepts (BoC) to understand how words with similar meanings can express different emotions. Their data for the work were retrieved from Twitter* as it has a lot of topics and slang words.
F.Ren et al.[7] have done a semi-automatic construction of youth slang with automatic Tag Annotation for 9 emotions (anger, fear, hate, joy, love, sorrow, surprise, neutral). They parsed the data from well-known Japanese youth blogs via
the Yahoo search engine. Authors used three methods to define emotion: 1) a dictionary-based method, 2) a machine learning-based method, and 3) using various features corpus based method. And as a result, they were able to increase the accuracy of the definition of emotion using their youth vocabulary.
Matsumoto et. al [5] suggested understanding the meaning of slang that can be changed over time by tracking the fluctuation of topics. They used the Latent Dirichlet Allocation method to generate a topic model for each month and analyzed
the transition of each similarity. And to calculate the similarity of topics, tf-idf (term frequency-inverse document frequency) was used, the tf-idf value is obtained by multiplying the frequency of occurrence of a word in a document by the number of documents in the corpus that contain this word.
For the Arabic language, Soliman et al. [8] have manually created slang sentiment words and idioms lexicon from comments (SSWIL) retrieved from Facebook. Their experience showed that by using SSWIL, they were able to obtain better performance in the classification of satisfied and unsatisfied comments. The Support Vector Machines classification technique has been used to achieve this goal.
Wilson et al. [9] in their paper introduced and released the first set of word embeddings trained on the content of Urban Dictionary, a crowd-sourced dictionary for slang words and phrases. Which is showed excellent results in a variety of common word embedding evaluations, starting from semantic similarity to word clustering tasks. They created a copy of Urban Dictionary in which each entry is represented as a paragraph with the headword, definition, examples, and tags as a separate sentence. Authors used fastText framework in order to train embeddings on Urban Dictionary.Wilson et al. [9] trained their models for 10 epochs using the skipgram architecture and used several parameters the publicly released fastText-CC embeddings: window size of 5, a negative sampling rate of 10, and a word-level dimensionality of 300. They achieved 64.4 % accuracy in sentiment classification on Twitter* dataset.
IV. METHODOLOGY
As mentioned earlier, we would like to use some of the methods suggested by Wu et al. Wu2018. We collected information about slang words from the newly created online slang dictionary in Kazakh ZhanaSozdik through the Python parser BeautifulSoup. But since this site was created not long ago, there is not much data to work with, although the site is updated every day. ZhanaSozdik also has an Instagram* page where the words differ from those on the site. And since Instagram is very popular in Kazakhstan, a special python parser will also be created to collect information from Instagram*. Additionally, we plan to expand the list of slang words manually.
We want to determine the polarity of words through their description. And for a better definition of semantics, we need to prepare the text. To do so, we first need to clean the description sentences from stop words and unnecessary noises like conjunctions, etc. Next, through the morphological analyzer based on Xerox finite-state tools for NLP. [2] identify the words that have polarity such as adjectives, etc. And the final step is to determine the polarity of the word using a sentimental analyzer [11] to build a dictionary. This will help in understanding the polarity of slang words. Also, by comparing the polarities of the descriptions of the words, it will be possible to determine the polarities of similar slangs.
The whole process is shown below in figure 1.
Figure 1. Steps in creation of Kazakh sentiment slang dictionary
V. RESULTS
For further work we consider an implementation of slang words dictionary to increase a text classification accuracy in determining the polarity of texts in sentiment analysis for Kazakh language. The new Kazakh sentiment slang dictionary may play a significant role for the future works in sentiment analysis in the Kazakh language and would be helpful in different NLP tasks related to Kazakh language
However, after thorough research of existing literature we faced an issue that there is not enough data of sentiment analysis for Kazakh language. Which creates limitations to obtain results at the present time.
In the future, we are planning to include a more detailed study of the topic and create the Kazakh sentiment slang dictionary itself by trying different methods and use it for sentiment analysis of people’s comments on social networks such as Facebook* or Twitter*.
References:
- Hady Elsahar and Samhaa R. El-Beltagy. “A fully automated approach for Arabic slang lexicon extraction from microblogs”. In: Lecture Notes in Computer Science (including subseries Lecture Notes in Artificial Intelligence and Lecture Notes in Bioinformatics) 8403 LNCS.PART 1 (2014), pp. 79–91. ISSN: 16113349. DOI: 10.1007/978-3-642-54906-9 7.
- Gulshat Kessikbayeva and Ilyas Cicekli. “Rule Based Morphological Analyzer of Kazakh Language”. In: (2015), pp. 46–54. DOI: 10.3115/v1/w14-2806.
- K. Manuel, Kishore Varma Indukuri, and P. Radha Krishna. “Analyzing internet slang for sentiment mining”. In: Proceedings - 2nd Vaagdevi International Conference on Information Technology for Real World Problems, VCON 2010 (2010), pp. 9–11. DOI: 10.1109/ VCON.2010.9.
- Kazuyuki Matsumoto et al. “Emotion recognition for sentences with unknown expressions based on semantic similarity by using Bag of Concepts”. In: 2015 12th International Conference on Fuzzy Systems and Knowledge Discovery, FSKD 2015 (2016), pp. 1394–1399. DOI: 10.1109/FSKD.2015.7382148.
- Kazuyuki Matsumoto et al. “Slang feature extraction by analysing topic change on social media”. In: CAAI Transactions on Intelligence Technology 4.1 (2019), pp. 64–71. ISSN: 24682322. DOI: 10.1049/ trit .2018. 1060.
- Wahyu Muliady and Harya Widiputra. “Generating Indonesian slang lexicons from twitter*”. In: Proceeding of 2012 International Conference on Uncertainty Reasoning and Knowledge Engineering, URKE 2012 (2012), pp. 123–126. DOI: 10.1109/URKE.2012.6319524.
- Fuji Ren and Kazuyuki Matsumoto. “Semi-Automatic Creation of Youth Slang Corpus and Its Application to Affective Computing”. In: IEEE Transactions on Affective Computing 7.2 (2016), pp. 176–189. ISSN: 19493045. DOI: 10.1109/TAFFC.2015.2457915.
- Taysir Hassan Soliman et al. “Sentiment Analysis of Arabic Slang Comments on Facebook*”. In: International Journal of Computers & Technology 12.5 (2014), pp. 3470–3478. DOI: 10.24297/ijct.v12i5.2917.
- Steven R Wilson et al. Urban Dictionary Embeddings for Slang NLP Applications. 2020, pp. 11–16. URL: https://fasttext.cc/.
- Liang Wu, Fred Morstatter, and Huan Liu. “SlangSD: building, expanding and using a sentiment dictionary of slang words for short-text sentiment classification”. In: Language Resources and Evaluation 52.3 (2018), pp. 839–852. ISSN: 15728412. DOI: 10.1007/s10579- 018-9416-0. arXiv: 1608.05129.
- Banu Yergesh, Gulmira Bekmanova, and Altynbek Sharipbay. “Sentiment analysis of Kazakh text and their polarity”. In: Web Intelligence 17.1 (2019), pp. 9–15. ISSN: 24056464. DOI: 10.3233/WEB-190396.
- Banu Yergesh et al. “Ontology-based sentiment analysis of kazakh sentences”. In: Lecture Notes in Computer Science (including subseries Lecture Notes in Artificial Intelligence and Lecture Notes in Bioinformatics) 10406 LNCS (2017), pp. 669–677. ISSN: 16113349. DOI: 10. 1007/978-3-319-62398-6 47.
*(social networks banned in the Russian Federation, Meta, recognized as extremist - ed.)