PROSPECTS FOR THE USE OF NEURAL NETWORKS IN THE EXAMINATION OF DRAFT LAWS
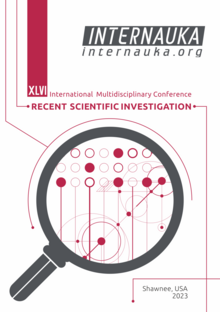
PROSPECTS FOR THE USE OF NEURAL NETWORKS IN THE EXAMINATION OF DRAFT LAWS
Nicolas Petrov
Master's student, Russian Presidential Academy of National Economy and Public Administration,
Russia, Moscow
ABSTRACT
Neural networks, have attracted considerable public attention for their ability to recognize complex patterns and make informed decisions. Analyzing complex legal frameworks, precedents, and legislative documents is a challenging task for lawyers. New techniques, such as neural networks, can improve the efficiency and accuracy of law-related tasks. Given the complex and evolving nature of legislation, the introduction of advanced technologies such as neural networks has the potential to help government agencies, lawyers, and researchers to comprehensively analyze and evaluate proposed legislation. This research article explores the prospects, potential benefits, and major challenges of using neural networks for legislative review, which could be an important step in the legislative process.
A neural network is a technique in artificial intelligence inspired by the structure and function of the human brain. It is a type of machine learning process called deep learning, which uses interconnected nodes or neurons in a layered structure resembling the human brain [1]. These models are designed to process complex patterns and relationships in data, allowing computers to learn from examples of input data and make predictions or decisions. Neural networks can be used for a variety of tasks such as image and speech recognition, natural language processing, anomaly detection, and many other tasks where it is important to capture complex patterns and relationships in data. Their ability to automatically learn and adapt allows them to solve problems that are difficult or impossible to solve with traditional approaches. Neural networks have the potential to revolutionize many industries, including the legal field.
A neural network usually contains the following layers of interconnected artificial neurons. The input layer which processes data, analyzes or classifies it and passes it on to the next layer. Hidden layers which receive input data from the input layer or other hidden layers. Each hidden layer analyzes the output data of the previous layer, processes it and passes it on to the next layer. And the output layer which gives the final result of all data processing by the artificial neural network [1].
Image 1. Neural network architecture [2]
Regardless, experts in the legal community unanimously believe that the legal profession is the most archaically organized and the least receptive to the advances of modern technology. Artificial Intelligence (AI) has long been effectively used in the legal profession. For example, neural networks are used to predict the outcome of cases, assess contractual risks, and even make judicial decisions. In China, judges are required to seek advice from artificial intelligence, with AI reviewing more than 100,000 cases every day and providing the court with rules of law to rule on, and the prosecutor's office in that eastern country is using a smart court to bring charges [3]. University College London (UCL), together with the Universities of Sheffield and Pennsylvania, were able to create an artificial intelligence complex. The AI has been taught to distinguish between "violation" and "no violation" in cases that the European Court of Human Rights has heard. The artificial intelligence correctly predicted the outcome of 584 cases. The accuracy rate is 79% [4].
Laws are the backbone of any legislative system, which may be developed as a future draft of a separate new law or for the purpose of amending and supplementing an existing law. The review of bills is a labor-intensive, time-consuming, and important process that often involves human experts. The ability to effectively summarize bills is critical to policymakers, lawyers, and citizens. Traditional methods of examining bills have traditionally relied on human expertise, which can be time-consuming, subjective and prone to bias. Using neural networks for automated bill analysis, in my opinion, could revolutionize the field, providing significant advantages such as speed, accuracy, scalability and cost-effectiveness. Neural networks can be trained to generate brief summaries, extracting important information from text. Techniques such as recurrent neural networks and long-term short-term memory networks have shown promising results in document abstracting tasks. Legal research requires extensive literature review and case studies, teaching neural networks to process vast amounts of legal texts, extract meaningful information, and identify legal precedents more effectively. Already, a private company, Luminance, has developed a system that uses a unique combination of supervised and unsupervised machine learning to find key information in thousands of documents [5].
Legislative review typically involves identifying potential conflicts with existing law, determining compatibility with constitutional principles, and assessing the feasibility and impact of proposed regulations. By integrating neural networks, these complex tasks can be automated, greatly reducing the time and effort required for manual analysis. This, in turn, speeds up the legislative process, allowing legislators to focus on the creative and qualitative aspects of drafting. Also, their use can provide automatic presumptive identification of public perceptions and the potential impact of bills on society. Therefore, the ability of neural networks to recognize patterns, process vast amounts of data, and make predictions makes them ideal candidates for legal analysis. Neural networks will be able to automate the vetting process by analyzing existing laws, relevant cases, and other legal texts, thereby providing lawmakers with valuable guidance during the drafting phase.
Thus, we can distinguish the following advantages of using neural networks in the examination of draft laws:
1. Neural networks can automate the analysis of laws, allowing the processing of large volumes of legal texts and reducing the time and effort required for interpretation and legal research;
2. Unlike human analysis, neural networks can provide a more objective and consistent interpretation of legal documents, minimizing the influence of personal biases;
3. Neural networks, in theory, will be able to capture complex legal concepts, understand their interrelationships, and identify the subtle nuances of legal language, facilitating a more comprehensive analysis.
While neural networks offer promising prospects for legislative expertise, several problems need to be addressed. These include the limited availability of training data, the transparency of neural network decision-making, and ensuring the accountability of automated systems. Transparency, especially in complex decisions made by neural networks, is, in my view, one of the biggest challenges on this list. Interpretability of their decisions is necessary to justify the results to stakeholders. Careful consideration of the quality of training data, biases, requires close attention to develop reliable and fair systems for analyzing bills. Research should focus on developing neural network models that provide interpretable explanations for their decisions, increasing transparency and allowing practitioners to understand and challenge the results. Also, it is worth noting the fact that laws evolve over time, and legal interpretation is a process with many nuances. Neural networks can have difficulty quickly adapting to changes in the legal framework or capturing the context-dependent nature of legal analysis. Legal language is often nuanced and context-dependent, making it difficult for neural networks to accurately understand and abstract. In addition, the interpretation of laws includes intangible aspects, such as social and moral values, which are difficult to translate into quantitative representations for neural networks.
Neural networks have significant potential to improve legislative review by lawyers, researchers, and government agencies. These advanced technologies offer numerous benefits, such as increased efficiency, and accurate analysis. Despite their potential, some challenges remain in using neural networks for law review. By solving the problems listed in this research paper, neural networks will assist legislators in identifying potential flaws, inconsistencies, and unintended consequences of proposed legislation. This analytical support will be able to help develop more effective legislation, minimizing unintended consequences and improving the overall quality of laws. Further research and collaboration between legal and artificial intelligence experts is needed to realize the full potential of neural networks for legislative review.
References:
- AWS. What Is A Neural Network? [Electronic resource]. URL: https://aws.amazon.com/what-is/neural-network/?nc1=h_ls;
- Chegg. Building the following network: [Electronic resource]. URL: https://www.chegg.com/homework-help/questions-and-answers/handwritten-digit-recognition-project-using-popular-mnist-database-collection-70000-handwr-q75864522;
- Daily Mail. China uses AI to 'improve' courts - with computers 'correcting perceived human errors in a verdict' and JUDGES forced to submit a written explanation to the MACHINE if they disagree [Electronic resource]. URL: https://www.dailymail.co.uk/news/article-11010077/Chinese-courts-allow-AI-make-rulings-charge-people-carry-punishments.html;
- PeerJ Computer Science. Predicting judicial decisions of the European Court of Human Rights: a Natural Language Processing perspective [Electronic resource]. URL: https://peerj.com/articles/cs-93/;
- Demystifying Luminance’s unsupervised machine learning [Electronic resource]. URL:https://www.luminance.com/news/blogs/20210409_luke_taylorsubjectmatterexpertluminance.html.